This post shows a setup that is able to run a backup server for daily backups at close to zero cost – both in terms of initial costs as well as running costs.
—
Backups are an annoying necessity of today’s life. Without them, loosing all your data in an instant is a realistic scenario. Sure, you might use cloudy stuff for your data that is (hopefully?) backed up but this comes with its own issues: Giving away your data, paying for the service, …
I, however, like to keep my data under my control. Therefore, I’d like to share my backup solution which might be useful for some of you – especially in these days of ever increasing energy prices.
Backup requirements
My backup system needed to fulfill the following requirements:
- Automated on a daily basis with snapshots for each day
- Encrypted
- Off-site
- Under my control
- Append only: Snapshots can only be added, never changed or deleted (to protect against ransomware attacks)
- Cheap to build and cheap to run
Many of these requirements can already be fulfilled by choosing the right backup software. I choose Restic, an in my opinion excellent piece of software for doing backups. It fixed the following requirements for me:
- Automated on a daily basis with snapshots for each backup: Since restic does automatic deduplication, doing daily snapshots does not hurt at all. I back up ~500k files with 500GB and typically each daily snapshot consumes a couple of KB + newly created data
- Encrypted: Restic encrypts backups by default
- Under my control: Restic is running on my hardware, using FOSS stacks
- Append only: Restic allows to use true append-only operation
So what I needed to fix by myself was “offsite” and “cheap to build and run”.
For the offsite requirement I decided to use some kind of machine that does not sit in my house. I considered both vServers as well as dedicated hardware.
A typical vServer that would be suitable for my needs would cost me around 15€ per month. Mh, so I would need to pay 180€ per year for something that I hopefully will never need… mmmh, nah, too little value for the money.
Next I considered running my own backup server in my garden shed (which has power but only wireless connectivity). This would cost me maybe 100€ of initial cost for the hardware (like a RPI or similar). And then, assuming a power consumption of 5W and 0.4€/kWh (yey, I live in Germany with the highest electricity costs worldwide) I would need to add another roughly 20€ per year for this device. So, yes, this seems okish but somehow I really don’t like the fact of having a RPI heating up my garden shed 24/7 while it is idling most of the time. In addition, RPIs are not exactly easy to get right now.
Since my garden shed does not have LAN, using techniques like Wake-On-LAN were also not possible. But luckily, I learned about a not very well known ACPI feature present in most machines: RTC wakeup. With this feature, you can instruct the real-time clock of your PC to wake up the machine at a specific time. Interestingly, this even works when the machine is turned off completely (not in standby or hibernate). With this, all my issues were solved: I can recycle a 15 year old laptop as a backup server and reduce its energy consumption to almost zero. This works as follows:
The RTC wakeup powers on the machine each day at 3am. My server that I want to back up notices the presence of the backup machine, starts a backup and after completion turns the backup machine off. Since Restic is blazingly fast (it takes 3 minutes to back up my 500k files, 500GB data), the machine only consumes its 7W for a duration of 3 minutes and the rest of the day 0W. This equals to 15mW on average, a power that you could even easily provide from a small solar cell in case you even do not have any power at all (think of a backup box just sitting in your garden).
How to use
The realization of such a system is very easy: On my data server I have a script that monitors the presence of the backup machine and if present, starts a backup and powers the machine back off. On the backup machine I just have an append-only restic server running.
This is the script running on my data server:
#!/bin/bash
# What should be backed up?
BACKUP_DATA="/data/"
NEXT_BACKUP="tomorrow 3am"
SERVER="backup-server-address" #ie 192.168.1.123
REST_USER="backup"
REST_PASSWORD="backup"
REPO_URL="rest:http://$REST_USER:$REST_PASSWORD@$SERVER/"
REPO_PASSWORD="backup"
SSH_USER="backup"
start_backup() {
export RESTIC_PASSWORD="$REPO_PASSWORD"
restic -r $REPO_URL backup $BACKUP_DATA
}
schedule_next_backup_and_power_off() {
echo "Shutting down server, will wake up again at $NEXT_BACKUP"
ssh $SSH_USER@$SERVER sudo rtcwake -m off -t $(date --date="$NEXT_BACKUP" +%s)
}
while true
do
if nc -z $SERVER 80 &> /dev/null; then
echo "Server online. Backing up..."
start_backup
schedule_next_backup_and_power_off
fi
sleep 5
done
The above script should be started on boot, for example by a SystemD service.
On my backup machine I simply run the restic server like so:
sudo docker run --env OPTIONS=--append-only -d -p 80:8000 -v /mnt/restic:/data --restart always --name rest_server restic/rest-server
# Add restic user after first start
docker exec -it rest_server create_user backup backup
Since the data server issues the rtcwake command on the backup server, we need to make sure that the access via ssh onto the backup server cannot do anything harmful (to not allow an attacker to circumvent the append-only nature of the setup). To do so, issue the following commands on the backup server:
sudo su
# Only allow restricted shell
usermod -s /bin/rbash backup
# Allow to run rtcwake
echo "ALL ALL=(ALL) NOPASSWD: /sbin/shutdown, /sbin/poweroff, /sbin/halt, /sbin/reboot, /usr/sbin/rtcwake" >> /etc/sudoers
In addition, you should add the public key from your data server to the authorized keys of the backup server:
# on your data server as root
ssh-copy-id backup@backup-server
Summary
A backup server made out of trashy old laptops, costing me 7ct/year to operate? Yes please! I am running this setup for a couple of weeks now and so far I am really really happy with it.

The Nokia communicator was quite expensive back when it came out and unfortunately, the devices still are today (since they became collectables).
Therefore, I built my own device, based on a Nokia 5110 brickphone:
Inside of the original battery I placed a custom designed micro Bluetooth keyboard:

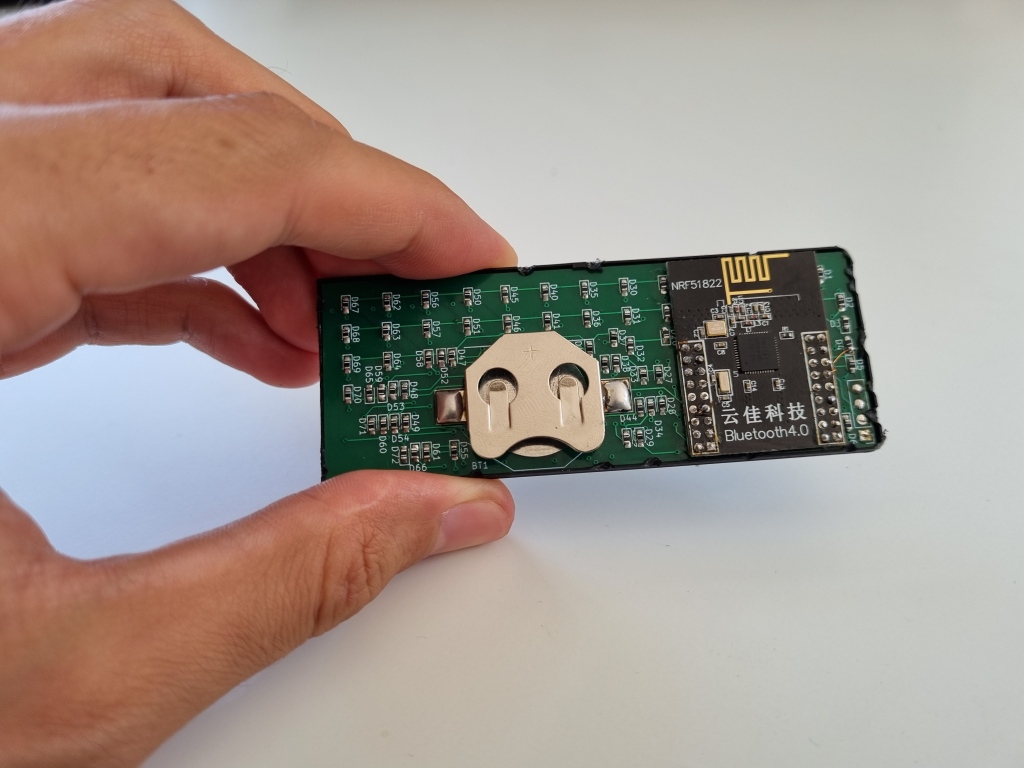

The front cover has been turned as well into a Bluetooth keyboard:
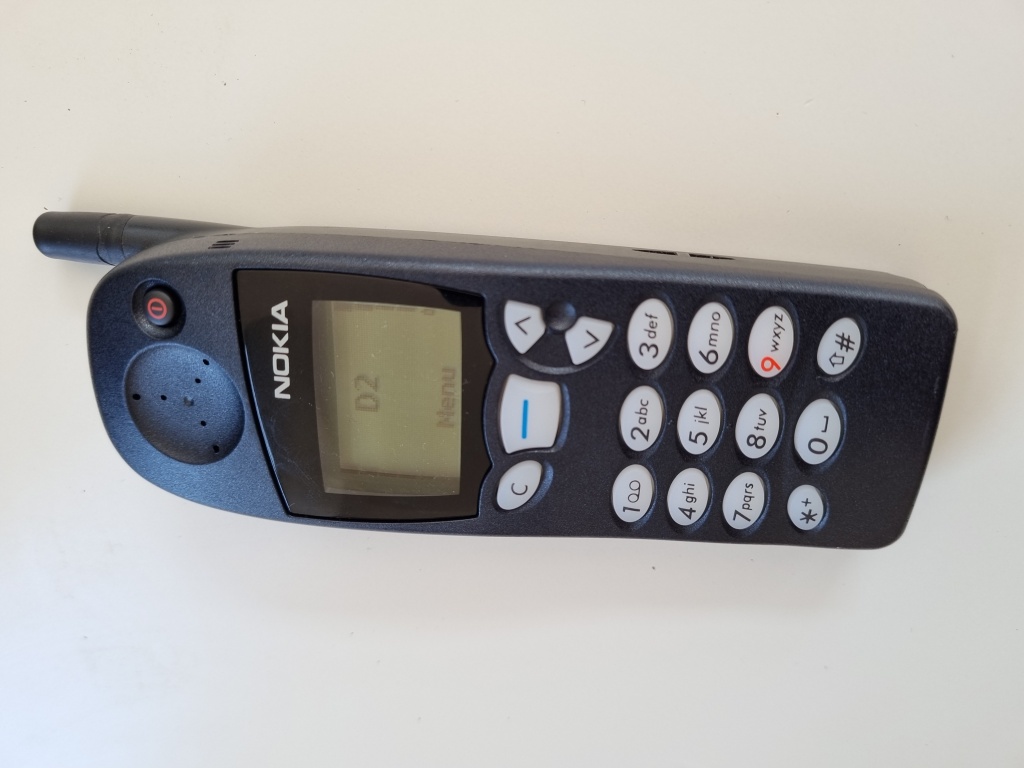
Design files can be found here:
PCB & Mechanics: https://github.com/befinitiv/nokia5110_communicator
Source code: https://github.com/befinitiv/ble_app_hid_keyboard_nokia

This post describes a simple DIY battery replacement that powers an oscilloscope multimeter from USB-C instead of batteries.
Recently, I bought an oscilloscope multimeter which is nice to have, but really eats away the batteries. Therefore, I built a replacement battery that powers the device from USB-C:

The Openscad design can be found here: https://gist.github.com/befinitiv/1568857c133cee344a5894d7f8c03d1b
This post presents an easy to use Youtube Music player that plays music from small token objects
—

The player presented in this post plays music based on Youtube URLs that are encoded as a QR tag and attached to “tag objects”.
If these objects are placed on top of the box, the music will get played immediately. You can see it in action here:
The source code as well as the schematics can be found in my Github: befinitiv/befibox
TLDR: Bought a Samsung S21 5G, it broke 3 times within warranty time, Samsung gave up repairing it and leaves me with a broken 700€ phone.
Last year my old phone broke and I replaced it with the Samsung top model because I expected it to give me the least trouble. The opposite was the case.
After a month the camera started to shake on its own, even when sitting on a tripod. Clearly, this was a malfunctioning image stabilization.
Next, the mainboard had a fault and the phone crashed and rebooted every minute or so.
Samsung repaired the faults so I was okish with them. Still, I was left for weeks without a phone and they did not provide me a replacement device during that time.
The phone worked ok for a couple of months but then started to break in a quite hilarious way: The actual phone functionality broke. During calls my microphone stopped working after approximately one minute and at the other end you would here nothing but nasty digital noise.
So I did the usual: I sent the phone to Samsung to repair it, they sat on it for weeks and finally returned it together with a letter that said:
Sorry, we cannot repair your device because we do not have any replacement parts for it.

So, Samsung, are you saying: We, the manufacturer of the device, do not have any replacement parts for our top of the line product, which is still in production?
It is really hard for me to decide which is the worst. That their best phone model broke 3 times within a couple of months? That this is a well known problem and they are unwilling to acknowledge it? That they used the silliest possible excuse to avoid repairing it? That they leave me with a phone that cannot do any calls? That I bought a 700€ Phone and they just show me the finger and leave me with a broken device?
So, if you as a customer read this, I hope that your conclusion is the same as mine: Do not buy Smartphones from Samsung. Their quality is laughable, they are dishonest about this fact and they do not care at all about you as a customer.
Have you ever been annoyed by having to charge your headphones? There is a cure for that 🙂
Let’s whack some weed 🙂